Hi there! My name is Thomas DeSilvio, and I am a biomedical engineering PhD student at Case Western Reserve University, Cleveland OH.
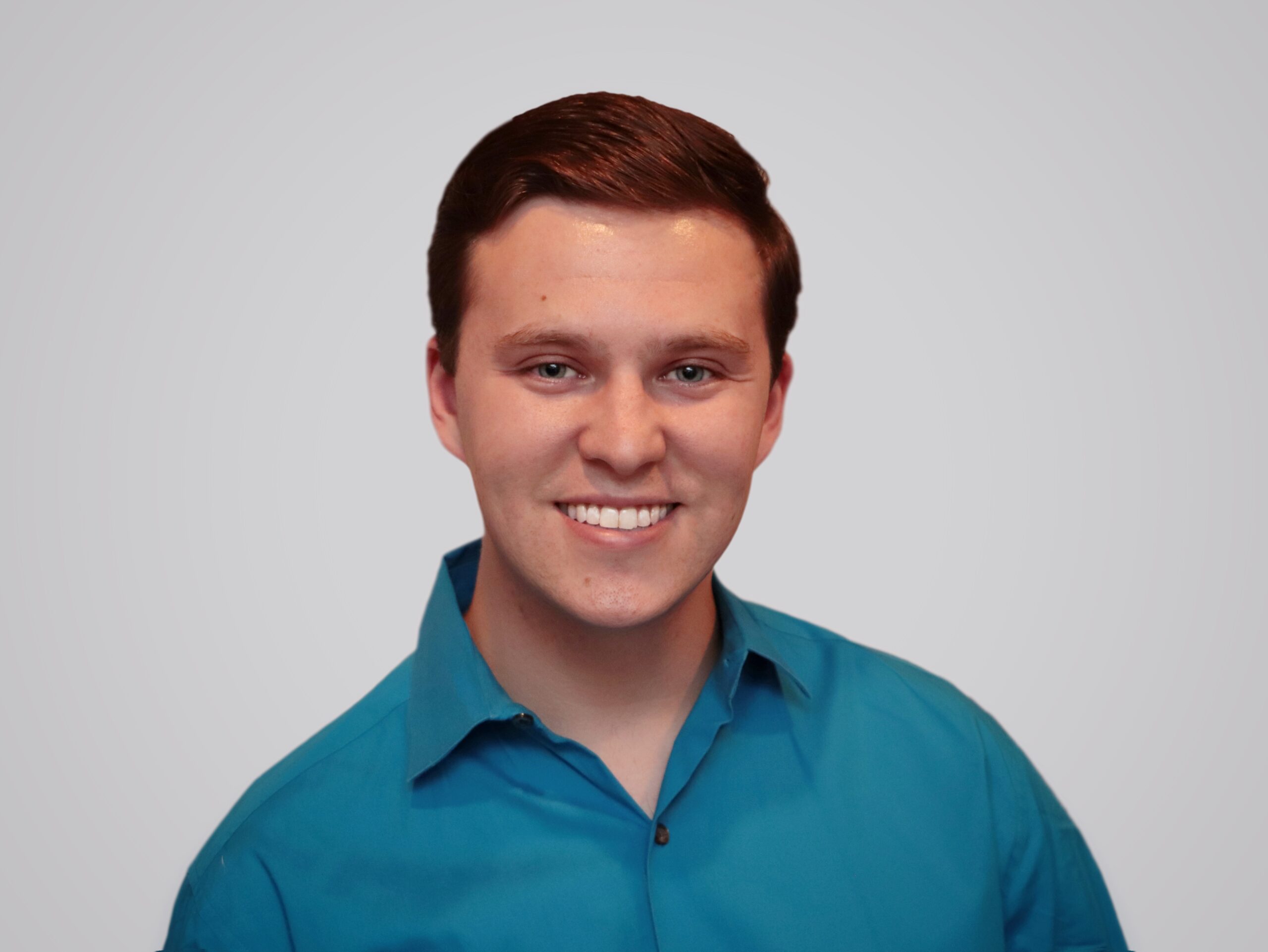
My Research
My research focuses on developing novel artificial intelligence, specifically machine learning and deep learning, tools for medical imaging analysis to improve computer-aided diagnosis and treatment evaluation. By applying these tools to magnetic resonance imaging (MRI) and digital pathology, I hope to enhance cancer detection, intervention, and treatment evaluation for patients around the world.
I work in the Imaging Informatics for Interventions (INVent) Lab and The Center for AI Enabling Discovery in Disease Biology (AID2B) at Case Western Reserve University, Cleveland OH.
Journal Publications
Fan F, Martinez G, DeSilvio T, Shin J, Chen Y, Wang W, Ozeki T, Lafarge M, Koelzer V, Barisoni L, Madabhushi A, Viswanath S.E, & Janowczyk A. (2023). CohortFinder: an open-source tool for data-driven partitioning of digital pathology and imaging cohorts to yield robust machine-learning models. npj Imaging 2, 15 (2024). https://doi.org/10.1038/s44303-024-00018-2
Alvarez-Jimenez C, Antunes J, Wei Z, DeSilvio T, Ismail M, Madabhushi A, Romero E, Tiwari P, Viswanath S.E. A novel STrUctural DEformation Orientation (STuDeO) Radiomic Descriptor for evaluating response to neoadjuvant therapy in rectal cancers via MRI. January 2025. npj Precision Oncology 2025 (under review)
Sadri AR, Azarianpour S, Chirra P, Singh S, DeSilvio T, Madabhushi A, Viswanath SE. Variability Regularized Feature Selection (VaRFS) for Optimal Identification of Robust and Discriminable Features from Medical Imaging. February 2025. npj Imaging. (under review)
Singh S, DeSilvio T , Sadri A, Labbad M, Gupta A, Bingmer K, Paspulati R, Gollamudi J, Friedman A, Liska D, Stein S, Marderstein E, PuryskoA, Krishnamurthi S, and Viswanath S.E., Radiomic tumor diversity features across pre- and post-chemoradiation MRI are associated with pathologic complete response to neoadjuvant chemoradiation in rectal cancers: a multi-institutional study (under preparation)
Conference Publications
Bao L, DeSilvio T, Parker BN, Hariri M, Chirra P, Tang S, O’Connor GM, Steinhagen E, Miller-Ocuin J, Gupta A, Carroll A, Crittenden M, Gough MJ, Krishnamurthi S, Young KH, Marderstein EL, Viswanath SE. Machine Learning Analysis of Image Features on Baseline MRI Can Identify Responders to Multiple Neoadjuvant Therapy Regimen in Rectal Cancers. Oral presentation at: Association of VA Surgeons Annual Meeting; April 8, 2024; Miami, FL. (oral presentation).
Parker B, Kong M, DeSilvio T, Bao L, Flannery B, Tang S, O’Connor G, Gupta A, Steinhagen E, Purysko A, Carroll A, Crittenden M, Gough M, Young K, Marderstein E, Viswanath S. Automated Annotation of Rectal Tumors on MRI Using Human-Informed Deep Learning. Oral presentation at: Association of VA Surgeons Annual Meeting; April 8, 2024; Miami, FL. (oral presentation).
DeSilvio T* and Kong M*, Bao L., Flannery B., Parker B. N., Tang S., O’Connor G., Gupta A., Steinhagen E., Purysko A. S., Marderstein E. L., Carroll A., Crittenden M., Gough M., Young K., Viswanath S. E.; Abstract 7386: Multi-plane rectal tumor segmentation on pre-treatment MRI via human-in-the-loop deep learning. Cancer Res 15 March 2024; 84 (6_Supplement): 7386. https://doi.org/10.1158/1538-7445.AM2024-7386 *indicates co-first authors (poster).
Flannery B., Hariri M., DeSilvio T., Sadri A., Nguyen J., Remer E. M., Krishnamurthi S., Viswanath S. E.; Abstract 7379: Deep learning based risk stratification of pre-operative CT scans is prognostic of overall survival in kidney cancers. Cancer Res 15 March 2024; 84 (6_Supplement): 7379. https://doi.org/10.1158/1538-7445.AM2024-7379 (poster).
Bao L., DeSilvio T., Parker B. N., Hariri M., Chirra P., Labbad M., Tang S., O’Connor G. M., Steinhagen E., Miller-Ocuin J. L., Gupta A., Marderstein E. L., Carroll A., Crittenden M., Gough M. J., Krishnamurthi S., Young K. H., Viswanath S. E.; Abstract 2582: Intra- and peri-tumoral radiomic features are predictive of pathologic response to multiple neoadjuvant therapy regimen in rectal cancers via pre-treatment MRI. Cancer Res 15 March 2024; 84 (6_Supplement): 2582. https://doi.org/10.1158/1538-7445.AM2024-2582 (poster).
DeSilvio T* and Kong M*, Bao L, Flannery B, Parker BN, Tang SM, O’Connor GM, Gupta A, Steinhagen E, Purysko A, Marderstain EL, Carroll A, Crittenden M, Gough M, Young KH, Viswanath SE. “Human-in-the-loop informed deep learning rectal tumor segmentation on pre-treatment MRI,” Proc. SPIE 12927, Medical Imaging 2024: Computer-Aided Diagnosis, 1292707 (3 April 2024); https://doi.org/10.1117/12.3008637 *indicates co-first authors (oral presentation).
Flannery F, DeSilvio T, Sadri AR, Hariri M, Remer EM, Nguyen J, Viswanath SE. “Spatial attention wavelon network (SpAWN) for survival-based risk stratification in kidney cancers via CT,” Proc. SPIE 12927, Medical Imaging 2024: Computer-Aided Diagnosis, 129272E (3 April 2024); https://doi.org/10.1117/12.3008727 (poster).
Le H, DeSilvio T, Patel S, Vasilyeva D, Pathak T, Friedman K A, Stein S L, Viswanath SE, “Computerized Pathomic Descriptors of Residual Tumor on Digitized Pathology Specimens for Evaluation of Tumor Stage and Regression Grade after Neoadjuvant Chemoradiation in Rectal Cancers”, ASCRS 2023 (poster).
Singh S, DeSilvio T, Sadri A, Labbad M, Bingmer K, Paspulati R, Friedman K A, Liska D, Stein S L, Marderstein E, Krishnamurthi S, Purysko A, Viswanath SE, “Tumor diversity features across pre- and post-chemoradiation MRI are associated with degree of pathologic response to chemoradiation in rectal cancers: a multi-institution study”, RSNA 2022 (poster).
Sadri A, DeSilvio T, Debnath T, Toth R, Bera B, Gupta A, Soman S, Nayate A, Hill V, Tiwari P, and Viswanath SE, “A light-weight deep learning web app for rapid identification of poor-quality structural MRI scans: A multi-institutional study”, RSNA 2022 (poster).
Sadri A, DeSilvio T, Debnath T, Toth R, Bera B, Gupta A, Soman S, Nayate A, Hill V, Tiwari P, and Viswanath SE, “Unsupervised identification of MRI artifacts via integration of deep learning and image quality measures”, RSNA 2022 (poster).
Sadri AR, DeSilvio T, Chirra P, Singh S, Viswanath SE, Residual Wavelon Convolutional Networks for Characterization of Disease Response on MRI. In: Wang, L., Dou, Q., Fletcher, P.T., Speidel, S., Li, S. (eds) Medical Image Computing and Computer Assisted Intervention – MICCAI 2022. MICCAI 2022. Lecture Notes in Computer Science, vol 13433. Springer, Cham. https://doi.org/10.1007/978-3-031-16437-8_35 (poster).
Skills
Python | MATLAB | Deep Learning | Computer Vision |
PyTorch | Keras | Tensorflow | Docker |
Github | Image Processing | High Performance/Distributed Computing | Medical Image Analysis |